![]() | ![]() |
Description:
The objective of the project was to propose hybrid machine-learning approaches to address supervision, predictive maintenance, and process optimization problems occurring in Industry 4.0. This project aimed at highlighting the synergies between machine learning approaches and model-based approaches. The benefits and limits of each approach were intended to be analyzed and the best of each approach to be used in the combinations (ensemble learning approaches). These combinations were intended to be exemplified in the context of Industry 4, using each partner in-house tools representative of each category of problem.
Members:
![]() | louise@laas.fr |
subias@laas.fr | |
echanthe@laas.fr | |
![]() | jsotom@pucp.edu.pe |
gustavo.perez@pucp.pe | |
![]() | jlaguilarc@eafit.edu.co |
emontoya@eafit.edu.co |
Postdoctoral student:
![]() |
ehsepulved@laas.fr |
![]() |
rjgarciah@eafit.edu.co |
Doctoral student:
![]() | lenciso@pucp.edu.pe |
![]() |
mljimenezn@eafit.edu.co |
Publications:
Link to the list
Results:
The objective has been implemented by carrying out a bibliometric review of the literature on the latest advances in industry 4.0 around automatic diagnosis, emphasizing methods based on hybrid model-based diagnosis (MBD) methods and data-based diagnosis (DBD) methods.
The following results have been obtained:
- Review the combinations of MBD and DBD existing in the literature in the context of Industry 4.0
- Identify the different frameworks to develop hybrid ML approaches to problems in Industry 4.0
- Present two in-house ML approaches that are applicable to diagnosis, namely LAMDA (Learning Algorithm for Multivariable Data Analysis), DyClee (Dynamic Clustering for Evolving Environments) and their variants, and discuss how they could be hybridated.
The systematic review was done following a simplification of the standard PRISMA 2020 (Page et al., 2021), which has been used in works as (Madhavan et al., 2022). This methodology has four phases: (i) identification of the need for revision, (ii) definition of a search protocol, (iii) carrying out the review, and (iv) analysis of the review.
Research questions were the following:
Q1: Which are the potential combinations between MBD and DBD in the context of Industry 4.0? (datasets/benchmarks and validation strategies)
Q2: Which are the different unified frameworks to integrate hybrid ML approaches that allow the reutilization of the solutions/algorithms for Industry 4.0 problems?
The methodology is illustrated in Figure 1.
Figure 1: The methodology followed in the bibliography search
Three main MBD and DBD hybridation architectures have been identified:
- Serial integration of methods,
- Parallel integration of methods,
- Ad-hoc integration.
An in-depth analysis of the methods associated with the various diagnostic tasks, namely residual generation, fault isolation, residual selection, fault detection, and system identification was performed and the roles of MBD versus DBD methods was identified as presented in Figure 3.
Figure 3: Roles of MBD and its relation with DBD methods. UVE stands for “Unknown Variable Elimination”, SA stands for “Structural Analysis, PS stands for “Parity Space”, PE stands for “Parameter Estimation”, OB stands for , STT stands for “Statistical Tools”, REG stands for “Regression”, CLU stands for “Clustering”, and CLA stands for “Classification”.
The various application domains for hybrid methods have been identified as in Figure 4 and the application works related to Industry 4.0 have been analysed.
Figure 4: Application areas of hybrid methods.
Finally, opportunities for hybridization of two in-house ML systems, namely DyClee and LAMDA, have been discussed.
Sponsors:
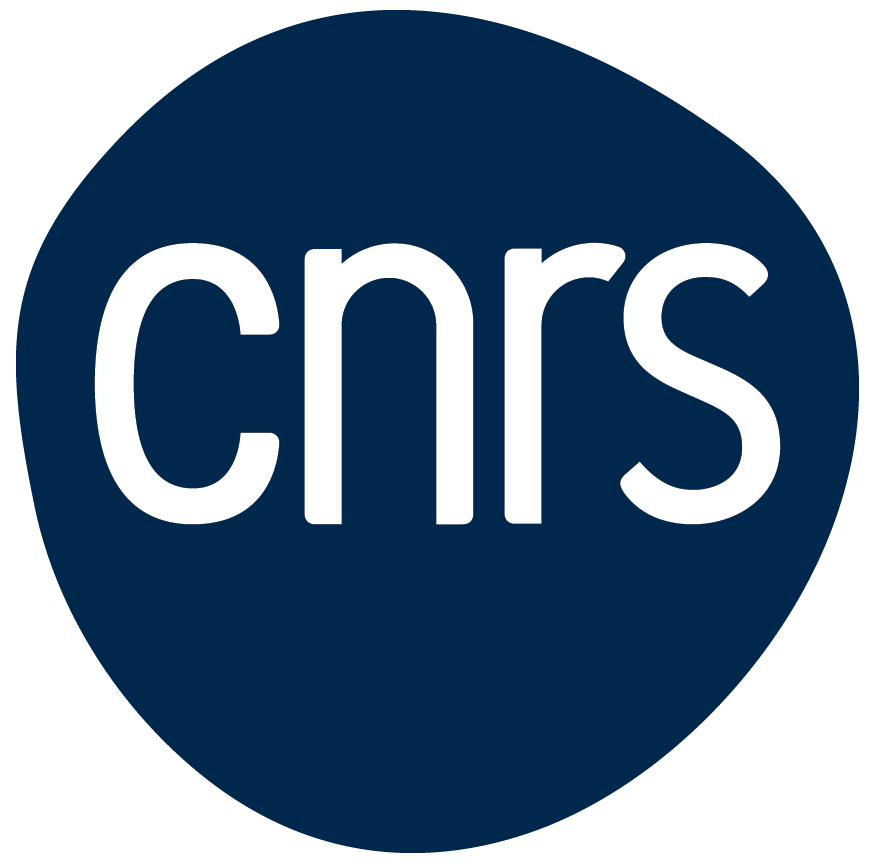
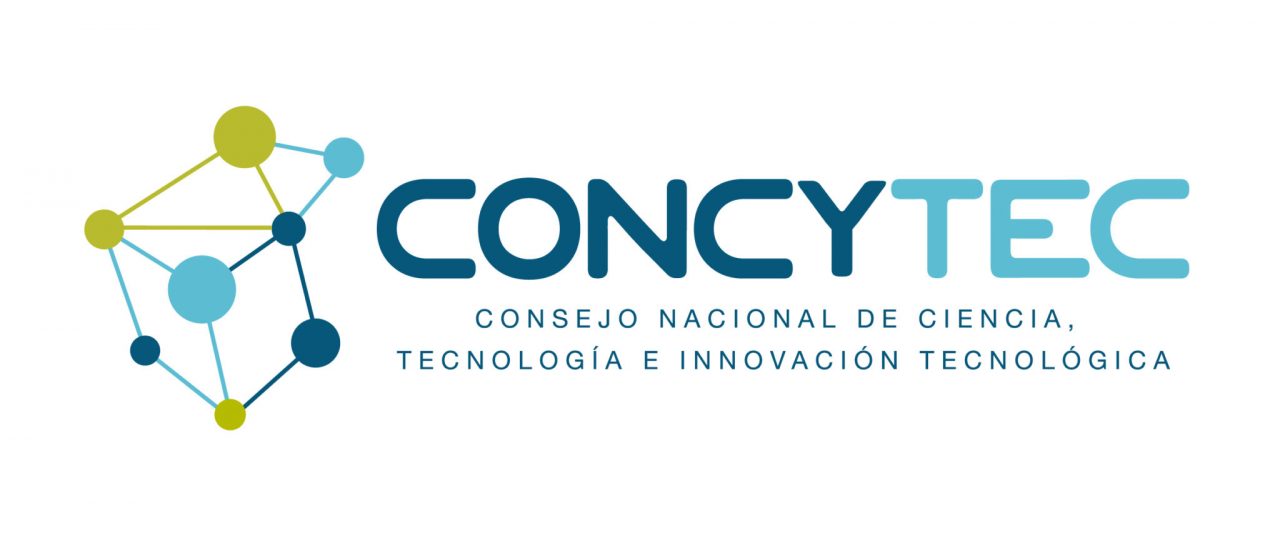
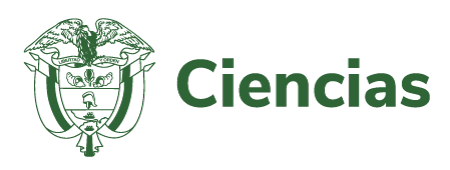